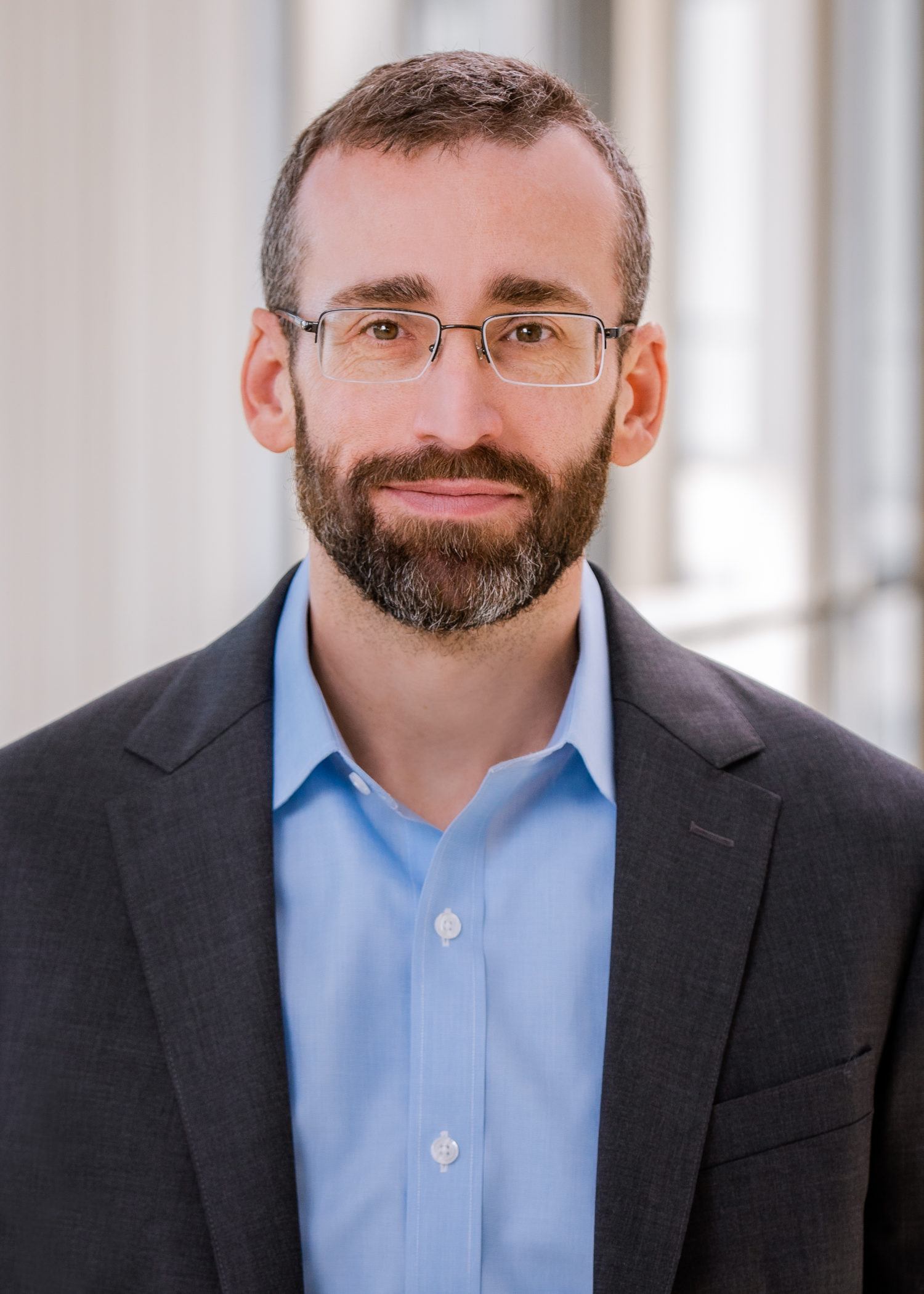
Since the onset of the COVID-19 pandemic, hospitals and health systems have pushed forward with innovative technology solutions with great expediency and proficiency. Healthcare organizations were quick to launch telehealth solutions and advance digital health to maintain critical patient relationships and ensure continuity of care. Behind the scenes, hospitals and health systems have been equally adept at advancing technology solutions to support and enhance clinical care delivery. This includes adopting clinical surveillance systems to better predict and prevent an escalation of the coronavirus.
Clinical surveillance systems use real-time and historical patient data to identify emerging clinical patterns, allowing clinicians to intervene in a timely, effective manner. Over time, these clinical surveillance systems have evolved to help healthcare organizations meet their data analytic, surveillance, and regulatory compliance needs. The adaptability of these systems is evidenced by their expanded use during the pandemic. Healthcare organizations quickly pivoted to incorporate COVID-19 updates into their clinical surveillance activities, providing a centralized, global view of COVID-19 cases.
To gain insight into the COVID-19 crisis, critical data points include patient age, where the disease was likely contracted, whether the patient was tested, and how long the patient was in the ICU, among other things. Surveillance is also able to factor in whether patients have pre-existing conditions or problems with blood clotting, for example. This data trail is helping providers create a constantly evolving coronavirus profile and provides key data points for healthcare providers to share with state and local governments and public health agencies. In the clinical setting, the data are being used to better predict respiratory and organ failure associated with the virus, as well as flag COVID-19 patients at risk for developing sepsis.
What’s driving these advancements? Clinical surveillance systems powered by artificial intelligence (AI). By refining the use of AI for clinical surveillance, we can proactively identify an expanding range of acute and chronic health conditions with greater speed and accuracy. This has tremendous implications in the clinical setting beyond the current pandemic. AI-powered clinical surveillance can save lives and reduce costs for conditions that have previously proven resistant to prevention.
Eliminating healthcare-associated infections
Despite ongoing prevention efforts, healthcare-associated infections (HAIs) continue to plague the US healthcare system, costing up to $45 billion a year. According to the Centers for Disease Control and Prevention (CDC), about one in 31 hospitalized patients will have at least one HAI on any given day. AI can analyze millions of data points to predict patients at-risk for HAIs, enabling clinicians to respond more quickly to treat patients before their infection progresses, as well as prevent spread among hospitalized patients.
Building trust in AI
While the benefits are clear, challenges remain to the widespread adoption and use of AI in the clinical setting. Key among them is a lack of trust among clinicians and patients around the efficacy of AI. Many clinicians remain concerned over the validity of the data, as well as uncertainty over the impact of the use of AI on their workflow. Patients, in turn, express concerns over AI’s ability to address their unique needs, while also maintaining patient privacy. Hospitals and health systems must build trust among clinicians and patients around the use of AI by demonstrating its ability to enhance outcomes, as well as the patient experience.
3 keys to building trust in AI
Building trust among clinicians and patients can be achieved through transparency, expanding data access, and fostering focused collaboration.
1. Support transparency
Transparency is essential to the successful adoption of AI in the clinical setting. In healthcare, just giving clinicians a black box that spits out answers isn’t helpful. Clinicians need “explainability,” a visual picture of how and why the AI-enabled tool reached its prediction, as well as evidence that the AI solution is effective. AI surveillance solutions are intended to support clinical decision making, not serve as a replacement.
2. Expand data access
Volume and variety of data are central to AI’s predictive power. The ability to optimize emerging tools depends on comprehensive data access throughout the healthcare ecosystem, no small task as large amounts of essential data remain siloed, unstructured, and proprietary.
3. Foster focused collaboration
Clinicians and data scientists must collaborate in developing AI tools. In isolation, data scientists don’t have the context for interpreting variables they should be considering or excluding in a solution. Conversely, doctors working alone may bias AI by telling it what patterns to look for. The whole point of AI is how great it is at finding patterns we may not even consider. While subject matter expertise should not bias algorithms,
it is critical in structuring the inputs, evaluating the outputs, and effectively incorporating those outputs in clinical workflows. More open collaboration will enable clinicians to make better diagnostic and treatment decisions by leveraging AI’s ability to comb through millions of data points, find patterns, and surface critically relevant information.
AI-enabled clinical surveillance has the potential to deliver next-generation decision-support tools that combine the powerful technology, the prevention focus of public health, and the diagnosis and treatment expertise of clinicians. Surveillance is poised to assume a major role in attaining the quality and cost outcomes our industry has long sought.
John Langton is director of applied data science at Wolters Kluwer, Health, where artificial intelligence is being used to fundamentally change approaches to healthcare. @wkhealth