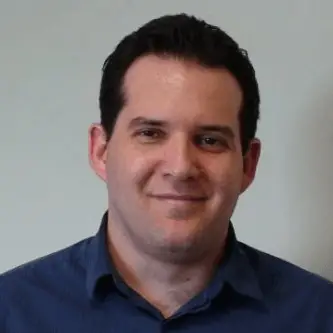
Few industries have embraced natural language processing (NLP) as openly as healthcare. With the ability to identify new variants of COVID-19 and help speed up clinical trials for the vaccine, the pandemic is just one example of what NLP is capable of achieving. And while new research points to NLP budgets growing significantly across vertical industries, locations, company sizes, and maturity levels, healthcare is leading the pack.
Big strides have been made in AI and NLP over the last year, but despite progress and increased investments, many of the challenges and barriers to entry remain the same.
The second annual NLP Industry Survey explores the triumphs, challenges, applications, and tools shaping NLP adoption.
The largest industry representation (17%) in this survey came from healthcare respondents, even greater than those in technology fields, which is reflective of overall industry adoption. As such, by analyzing how NLP has evolved over the last year in the healthcare space, we can get a glimpse of what’s on the horizon for the technology.
NLP Budgets Keep Growing
While 60% of tech leaders indicated their NLP budgets grew by at least 10%, a majority of healthcare technologists are spending 10-30% more on NLP compared to last year. It’s encouraging to see that even in the wake of the pandemic, IT investments in areas like NLP were still strong. It’s even possible that the circumstances of last year proved how valuable the technology can be.
For example, NLP algorithms are now able to generate protein sequences and predict virus mutations, including key changes that help the coronavirus evade the immune system, according to MIT research. Kaiser Permanente uses NLP for extracting key features from EHR notes to optimize hospital patient flow — something critical to operations when healthcare organizations are overwhelmed with an influx of patients with differing levels of severity. These are just a few examples of what investments in NLP can achieve.
NLP Use Cases are Expanding
Aligned with respondents from other industries, healthcare tech leaders cited named entity recognition (NER) and document classification as the primary use cases for NLP. Looking ahead, we can expect growth in Q&A and natural language generation use cases powered by large language prediction models and related open-source alternatives. This will bring a greater level of humanity to NLP, as users will be able to speak in plain language directly to the technology and get a prompt, contextually relevant response.
De-identification is another use case that’s popular among highly regulated industries, such as healthcare. This enables users to redact personally identifiable information — names, addresses, social security numbers — subject to regulations like HIPAA and GDPR. De-identification will likely gain steam as a use case for other industries as businesses develop better data privacy practices. De-identification can also remove certain types of spurious correlations or biases from models, so will likely become more commonplace as Responsible AI practices become mainstream.
NLP Challenges: Accuracy Above All
When dealing with patients and their care, it’s clear why accuracy is the top priority users consider when evaluating an NLP solution. That said, it’s also one of the biggest challenges users face — 44% of them to be exact. Accuracy refers to the effectiveness of pre-trained models that come with NLP libraries, and it’s critical as results from previous tasks and models are used downstream.
Not only is getting it right from the get-go paramount but being able to tune models over time is equally important in order to prevent degradation and understand domain-specific jargon. As healthcare is an industry with unique challenges and nuances, this often requires a data scientist as well as a domain expert for optimal results. Because of the changing nature of data, regulations, and discoveries, even as NLP technology matures, accuracy will likely remain a challenge in years to come.
NLP Tools: Libraries and Cloud Use
Among the NLP libraries in use, Spark NLP remains the most popular. It is used by nearly a third (31%) of general respondents and 59% of healthcare respondents. Additionally, the use of NLP cloud services is rising steadily, with a 23% increase for the Top 4 cloud providers — AWS, Azure, Google, and IBM — since 2020. Even so, there are serious concerns by survey respondents about the pricing models for these cloud services as NLP practices scale. For solutions that need to process many documents on a regular basis, these cloud services are perceived as prohibitively expensive.
While progress has endured the global pandemic, a worldwide shortage of AI talent, and ongoing concerns about data sharing and privacy, NLP has proven its here to stay. Although it’s likely that challenges such as accuracy, scalability, and cost will persist into the future, new exciting use cases and advances in the technology will be interesting to watch, with the healthcare industry forging the path forward.
About David Talby
David Talby, Ph.D., MBA, is the CTO of John Snow Labs. the AI and NLP for healthcare company provides state-of-the-art software, models, and data to help healthcare and life science organizations put AI to good use. He has spent his career making AI, big data and data scientists solve real-world problems in healthcare, life science and related fields.